Unlocking the Power of Image Annotation Tools for Machine Learning
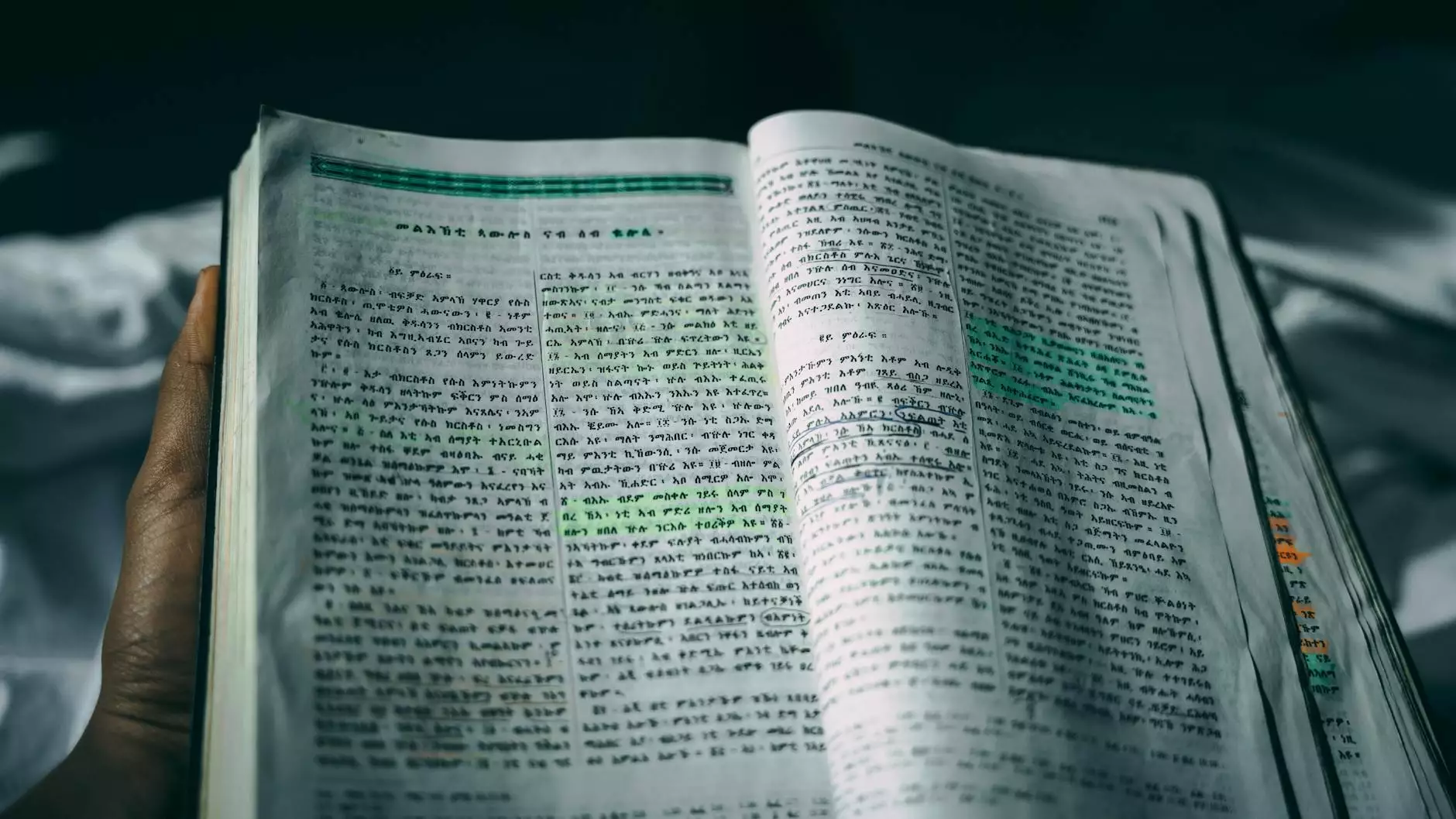
In the rapidly evolving landscape of artificial intelligence and machine learning, the significance of image annotation tools for machine learning cannot be overstated. These tools play a pivotal role in the training of AI models, enabling them to interpret and analyze visual data with remarkable accuracy. In this detailed article, we will explore the various aspects of image annotation tools, their applications in machine learning, and why choosing an effective data annotation platform like Keylabs.ai is critical for businesses today.
What is Image Annotation?
Image annotation is the process of labeling images to facilitate machine learning algorithms in understanding visual inputs. It involves adding useful metadata to images, allowing systems to recognize objects, features, or elements within those images. The labeled images are then used to train machine learning models, significantly enhancing their predictive capabilities.
The Importance of Image Annotation in Machine Learning
Machine learning models rely heavily on data for training, and annotated images serve as the primary source of supervised learning. Here are some crucial reasons why image annotation is essential:
- Supervised Learning: Without labeled data, supervised learning algorithms lack the guidance needed to learn from input features.
- Improving Model Accuracy: High-quality annotations lead to better model performance, reducing error rates significantly.
- Facilitating Complex Tasks: Tasks such as object detection, image segmentation, and classification depend greatly on precise annotations.
- Data Diversity: Annotated datasets provide diverse samples, helping models generalize better across different scenarios.
Common Types of Image Annotation Techniques
There are several techniques used for image annotation, and each serves unique purposes based on the requirements of the machine learning task:
1. Bounding Box Annotation
This method involves drawing a rectangular box around the objects of interest within an image. It's widely used in object detection tasks.
2. Semantic Segmentation
Semantic segmentation goes a step further by assigning a label to every pixel in an image, rather than just the object boundaries. This technique is crucial for applications that require precise understanding of image contents.
3. Polygon Annotation
For irregularly shaped objects, polygon annotation is employed, allowing annotators to provide a more accurate outline of an object’s boundaries.
4. Landmark Annotation
Landmark annotation involves marking specific points of interest in images, which is beneficial in facial recognition and medical imaging tasks.
Choosing the Right Image Annotation Tool
When it comes to selecting the best image annotation tool for machine learning, several factors should be considered:
- User Interface: A user-friendly interface enhances productivity and reduces the learning curve for annotators.
- Collaboration Features: Tools that facilitate teamwork streamline the annotation process, especially for large projects.
- Integration Capabilities: The ease with which the tool integrates with existing machine learning workflows is vital.
- Quality Control Mechanisms: Efficient tools should offer methods to verify and validate annotations, ensuring high accuracy.
- Scalability: The ability to handle large datasets and high volumes of images without compromising speed or quality.
Benefits of Using Keylabs.ai for Image Annotation
Keylabs.ai offers a comprehensive data annotation platform specifically designed to enhance the efficiency and accuracy of image annotation processes. Here are some of the benefits of utilizing Keylabs.ai:
1. High-Quality Annotations
Keylabs.ai ensures that all annotations are performed by trained professionals who understand the nuances of different annotation techniques. This contributes to superior model training.
2. Advanced Tools and Features
The platform provides a variety of tools and features including bounding boxes, polygons, and segmentation, enabling users to handle a wide range of annotation tasks effectively.
3. Fast Turnaround Times
With streamlined processes and a dedicated team, Keylabs.ai delivers quick turnaround times without compromising on quality, ensuring your machine learning projects stay on schedule.
4. Robust Quality Assurance
Keylabs.ai implements stringent quality control measures, including peer reviews and automated checks, to maintain high standards in data quality.
5. Integrative API
The API offered by Keylabs.ai allows seamless integration with existing workflows, making data transfer and processing smoother and more efficient.
Applications of Image Annotation in Various Industries
Image annotation is not limited to one sector; its applications are vast, influencing numerous industries:
1. Healthcare
In the medical field, image annotation is crucial for tasks like disease diagnosis and medical imaging analysis. Accurate annotations help in developing tools for automated image analysis.
2. Automotive
Self-driving cars utilize image annotation for object detection and recognition, enabling the vehicles to navigate safely in complex environments.
3. Retail and E-commerce
Image annotation aids in visual search functionalities, helping customers find products based on images, enhancing the shopping experience.
4. Agriculture
Farmers use annotated images for crop monitoring and health assessment, improving yield prediction and efficient farming practices.
5. Surveillance and Security
In security, annotated images support facial recognition systems and motion detection, enhancing safety protocols across various premises.
The Future of Image Annotation Tools
The future of image annotation tools for machine learning looks promising, with advancements in technology driving innovations. Key trends include:
1. Automation and AI Integration
As machine learning and AI technologies evolve, we can expect more automated annotation tools capable of self-learning and improving their accuracy over time.
2. Enhanced Collaboration Tools
Future annotation platforms will likely place a greater emphasis on collaboration, allowing teams to work on projects concurrently with enhanced communication features.
3. Cross-Platform Compatibility
Tools that can seamlessly operate across different devices and platforms will become increasingly important, especially in our mobile-centric world.
4. Focus on Security and Data Privacy
As data sensitivity grows, future tools will need to enhance security and ensure compliance with regulations to protect user information.
Conclusion
In conclusion, the use of image annotation tools for machine learning is indispensable for the development of accurate and efficient AI models. At Keylabs.ai, we are dedicated to providing the best data annotation services to cater to your machine learning needs. Our robust platform is designed to handle all aspects of image annotation while ensuring quality, speed, and ease of use. Adopt the future of machine learning with Keylabs.ai and experience the transformative power of precise data annotation.
For more information on our services and to get started with your image annotation projects, visit Keylabs.ai.